[ad_1]
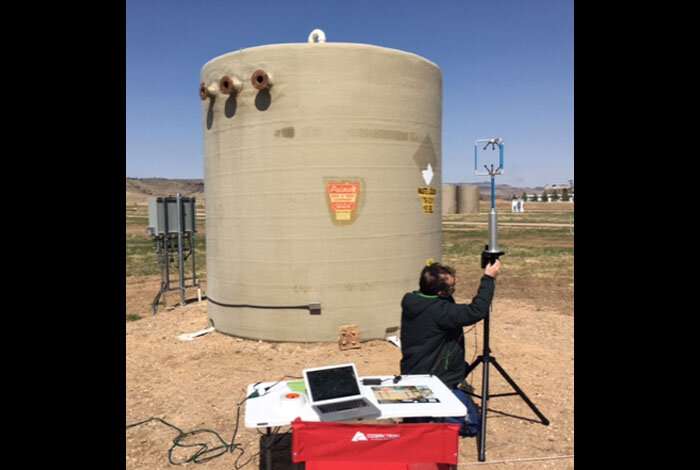
ALFaLDS is used during blind testing at the Oil and Gas Model Test Facility in Fort Collins, Colorado. Credit: Los Alamos National Laboratory
A new study confirms the success of a natural gas leak detection tool pioneered by scientists at Los Alamos National Laboratory that uses sensors and machine learning to locate leak points in oil and gas fields, promising new automatic and cost-effective sampling through extensive natural gas infrastructure.
“Our automated leak detection system quickly detects gas leaks, including small ones from failing infrastructure, and reduces costs as current methods of repairing gas leaks are time-consuming, expensive and slow,” said Manvendra Dubey , scientist and co-author of the Los Alamos National Laboratory. of the new study. “Our sensors have outperformed competitive techniques in terms of sensitivity to methane and ethane detection. In addition, our neural network can be coupled to any sensor, which makes our tool very powerful and will enable market penetration.”
ALFaLDS (Autonomous, Low Cost, Fast Leak Detection System) was developed to detect accidental releases of methane, a potent greenhouse gas, and won the 2019 R&D 100 award. ALFaLDS detects, locates and quantifies a leak of natural gas based on real-time measurements of methane and ethane (in natural gas) and atmospheric wind which are analyzed by a machine learning code trained to locate leaks. The code is trained using Los Alamos National Laboratory’s high-resolution plume dispersion models, and the training is refined on-site using controlled releases.
Test results using blind releases at an oil and gas well facility at Colorado State University in Fort Collins, Colorado, showed that the ALFaLDS accurately identifies engineered methane leaks and quantifies their size. This new ability to locate leaks with high skill, speed and accuracy at a lower cost promises new automatic and cost-effective sampling of fugitive gas leaks in wells and oil and gas fields, the paper in the newspaper Atmospheric environment: X concludes.
ALFaLDS ‘success in identifying and quantifying methane leaks in natural gas plants could lead to a 90% reduction in methane emissions if implemented by industry.
ALFaLDS used a small sensor, making it ideal for use on cars and drones. The Los Alamos team is developing the sensors that were integrated with a mini 3-D sonic anemometer and powerful machine learning code in these studies.
However, the code is self-contained and can read data from any gas and wind sensor to help quickly find leaks and minimize fugitive emissions from the vast natural gas extraction, production and consumption network.
With this integration, ALFaLDS offers a revolutionary approach for oil and gas service providers to leak detection, non-profit organizations investigating the problem, and national laboratories and academia researching natural gas production.
Team using machine learning drones to automate methane leak detection
Bryan Travis et al, neural networks to locate and quantify natural gas leaks for a MIR detection system, Atmospheric environment: X (2020). DOI: 10.1016 / j.aeaoa.2020.100092
Provided by Los Alamos National Laboratory
Quote: Machine Learning Driven Sensors Quickly Detect Gas Leaks (2020, Oct 29) Recovered Oct 29, 2020 from https://phys.org/news/2020-10-sensors-driven-machine-sniff- out-gas.html
This document is subject to copyright. Aside from any conduct that is correct for private study or research purposes, no part may be reproduced without written permission. The content is provided for informational purposes only.
[ad_2]
Source link