[ad_1]
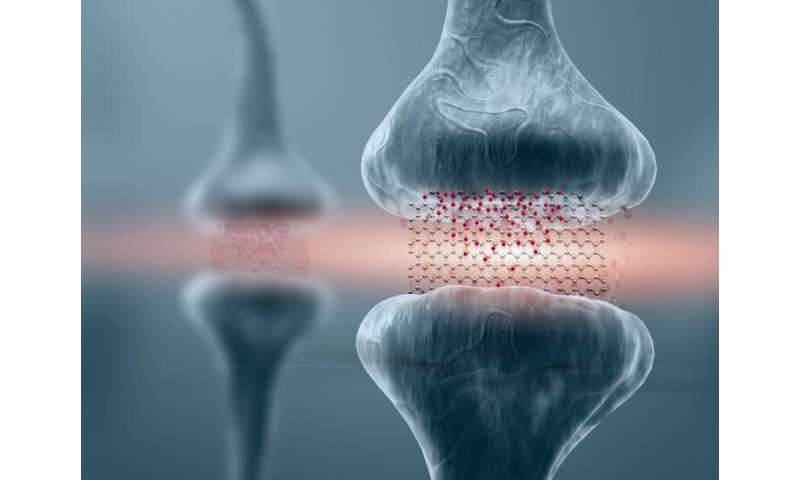
Graphene memristors open the door to biomimetic computation. Credit: Jennifer M. McCann / Penn State
With the slowing of progress in traditional computing, new forms of processing are coming to the fore. At Penn State, a team of engineers is attempting to pave the way for a type of processing that mimics the efficiency of the brain’s neural networks by exploiting the analog nature of the brain.
Modern computing is digital, made up of two states, on-off or one and zero. An analog computer, like the brain, has many possible states. It’s the difference between turning a light switch on or off and turning on a dimmer switch for a varying amount of lighting.
Neuromorphic or brain-inspired computing has been studied for more than 40 years, according to Saptarshi Das, the team leader and assistant professor of engineering and mechanics at Penn State. The novelty is that as the limits of digital computing have been reached, the need for high-speed image processing has grown, for example for self-driving cars. The rise of big data, which requires types of pattern recognition for which brain architecture is particularly suited, is another driving force in the pursuit of neuromorphic computation.
“We have powerful computers, there’s no question about that, the problem is you have to store the memory in one place and do the computation somewhere else,” said Das.
Moving this data from memory to logic and back takes a lot of energy and slows down processing speed. Also, this computer architecture requires a lot of space. If computation and memory storage could be in the same space, this bottleneck could be eliminated.
“We are creating artificial neural networks, which seek to emulate the energy and area efficiency of the brain,” explained Thomas Shranghamer, a doctoral student in the Das group and first author of a paper recently published in Nature Communications. “The brain is so compact it can fit over your shoulder, while a modern supercomputer takes up space the size of two or three tennis courts.”
Like the synapses connecting neurons in the brain that can be reconfigured, the artificial neural networks the team is building can be reconfigured by applying a short electric field to a sheet of graphene, the atomic-thick layer of carbon atoms. In this work they show at least 16 possible memory states, in contrast to the two in most oxide-based memristors or memory resistors.
“What we have shown is that we can control a large number of memory states with precision using simple graphene field effect transistors,” said Das.
The team believes it is feasible to bring this technology to commercial scale. With many of the largest semiconductor companies actively pursuing neuromorphic computing, Das believes they will find this work interesting.
In addition to Das and Shranghamer, the additional author of the article, titled “Graphene Memristive Synapses for High Precision Neuromorphic Computing,” is Aaryan Oberoi, a doctoral student in engineering and mechanics.
The brain-inspired electronic system could significantly reduce the carbon footprint of AI
Schranghamer, TF, Oberoi, A. & Das, S. Graphene memristive synapses for high-precision neuromorphic computation. Nat Common 11, 5474 (2020). doi.org/10.1038/s41467-020-19203-z
Provided by Pennsylvania State University
Quote: Graphene-Based Memory Resistors Show Promising Results for Brain-Based Computing (2020, Oct 29) Retrieved Oct 29, 2020 from https://phys.org/news/2020-10-graphene-based-memory-resistors -brain-based. html
This document is subject to copyright. Aside from any conduct that is correct for private study or research purposes, no part may be reproduced without written permission. The content is provided for informational purposes only.
[ad_2]
Source link